The discovery and development of new drugs is usually a long, expensive process, but recent advances in machine learning and artificial intelligence are starting to change that. By partnering with the Accelerating Therapeutics for Opportunities in Medicine (ATOM) consortium to create a new training experience, Butler University is preparing Pharmacy students for the future of drug discovery.
This past summer, five students in Butler’s Doctor of Pharmacy (PharmD) program participated in remote internships with ATOM—a global consortium with the goal of blending healthcare and computer science to create a faster drug discovery process. Starting with a coding boot camp led by Butler Assistant Professor Caleb Class, then working on individual research projects alongside ATOM mentors, students learned to integrate data science with their existing pharmacy expertise.
The interns worked to analyze, build, and curate data sets that can be used to advance ATOM’s open-source drug discovery platform. While most of them had little experience with machine learning prior to the program, they are excited to apply what they’ve learned to their pharmacy careers.
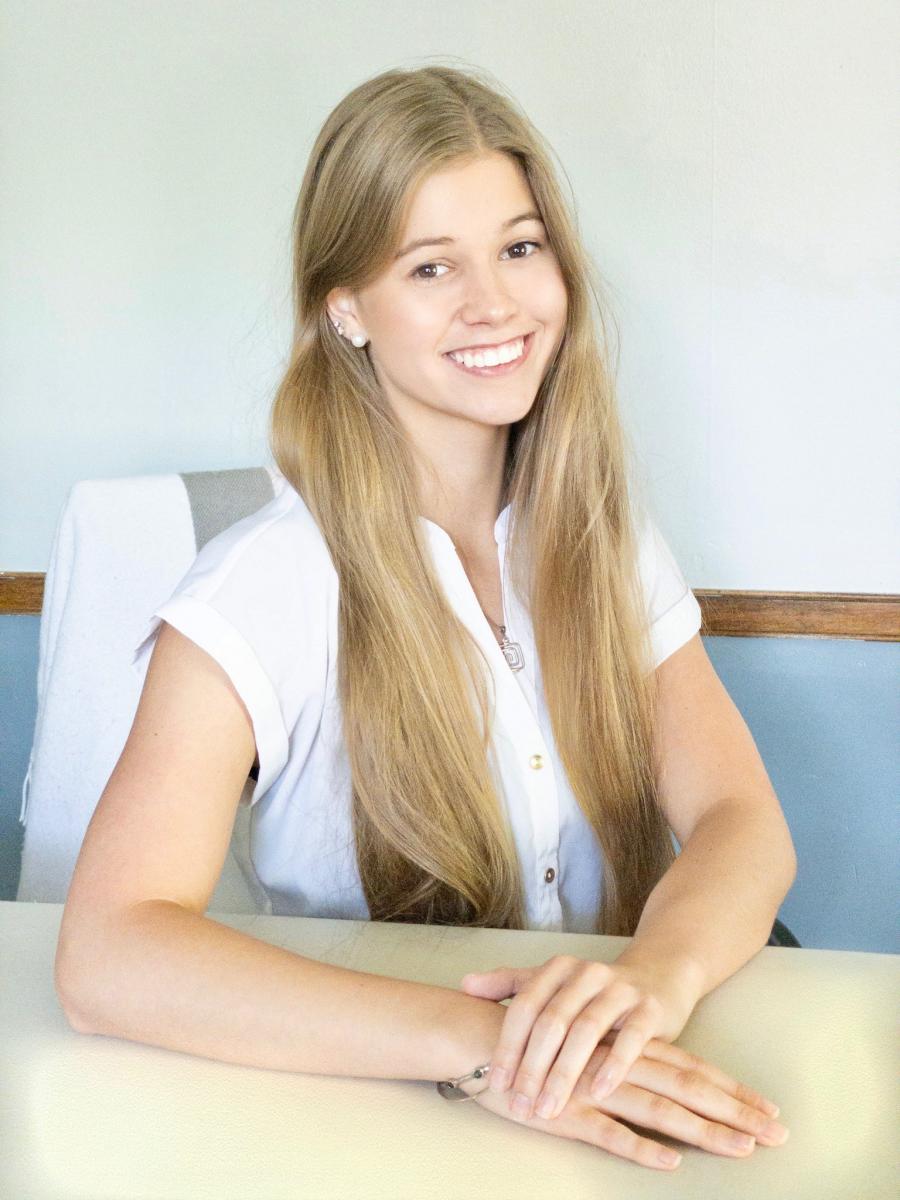
Paige Cowden (P2)
Project: “Data Curation for a Mitochondrial Membrane Potential Model”
Why did you pursue pharmacy?
I wanted to work in a hospital, but I didn’t want to be a doctor or a nurse, so I thought pharmacy might be cool. Also, addiction to prescription medications has affected people close to me, so I wanted to learn about drugs and be able to counsel people properly to prevent this from affecting others
What fascinates you most about the relationship between pharmacy and data science?
While learning to code was pretty difficult and frustrating at times, my knowledge of biology and science made it easier to compare the data I was working with. I could see how valuable my prior knowledge was to understanding the data, even though I was brand new to coding. It made me excited because I could see how machine learning could be used in my future career.
What have you learned from this internship opportunity?
Even if you’re bad at something, do it anyway. I wouldn’t say I became the most proficient at coding and analyzing data, but I definitely improved a lot. I think it’s frustrating trying something new for the first time, but keeping an open mind and not being so hard on yourself when you fail is key to becoming successful at something.
***
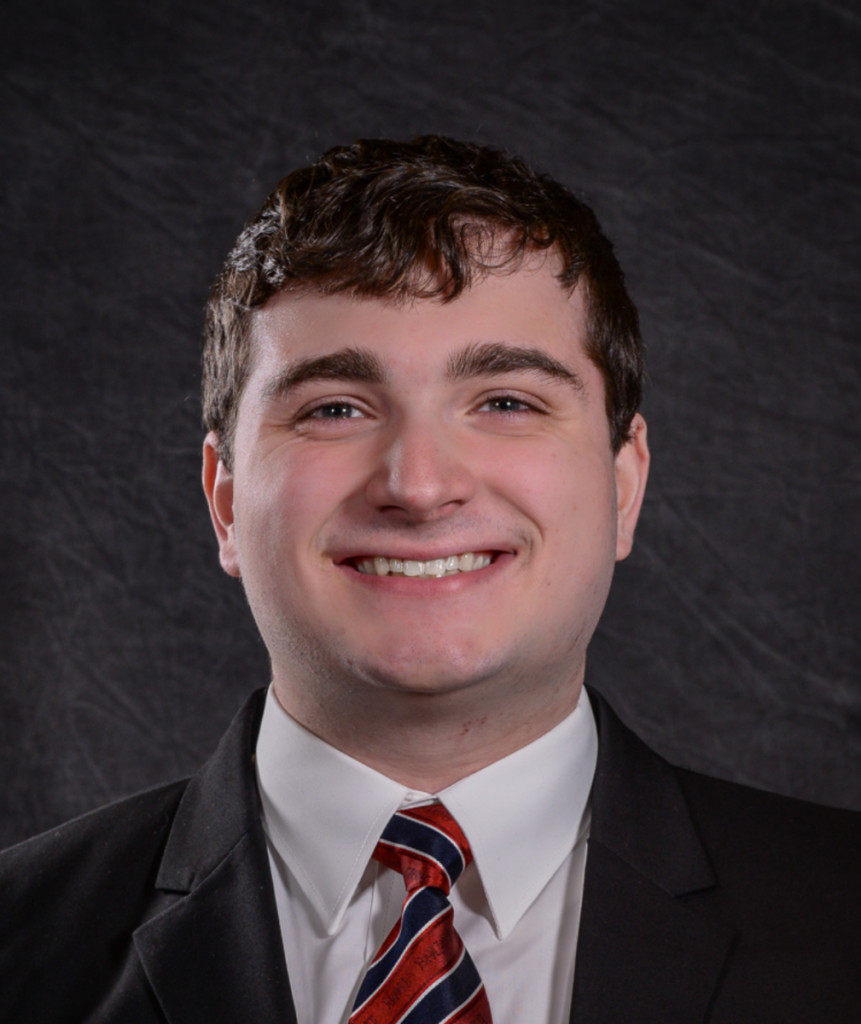
Chris Zeheralis (P3)
Project: “Open Cancer and Infectious Disease Datasets”
Why did you pursue pharmacy?
Pharmacy never really came across my radar until late in high school. I became a huge chemistry lover and enjoyed the idea of applying chemical concepts in a usable, practical setting, and in a way that could have a direct impact on people’s lives. I’ve always aimed to use my passions and skills to improve the world around me, and pharmacy just seemed like it could give me the platform to bring the change I’ve always desired.
What appealed to you about the ATOM internship?
I have always been fascinated with the power of computing, and I understand the inevitability of skills like programming and machine learning being incorporated into the healthcare field. I had attempted to teach myself how to code to no real avail. The ATOM internship allowed me the opportunity to learn coding in a more structured manner, connecting me with experts and professionals in multiple fields. I could also immediately apply what I was learning to something that had the potential to carry real weight outside of mere practice.
What did you learn from this experience?
Machine learning truly is the future of drug discovery. The sheer speed of methods like the ATOM Modeling PipeLine (AMPL) in discovering potential leads for molecule design, compared to the traditional methods, is astounding. This whole experience made me wish I had learned programming and coding at an earlier age.
***
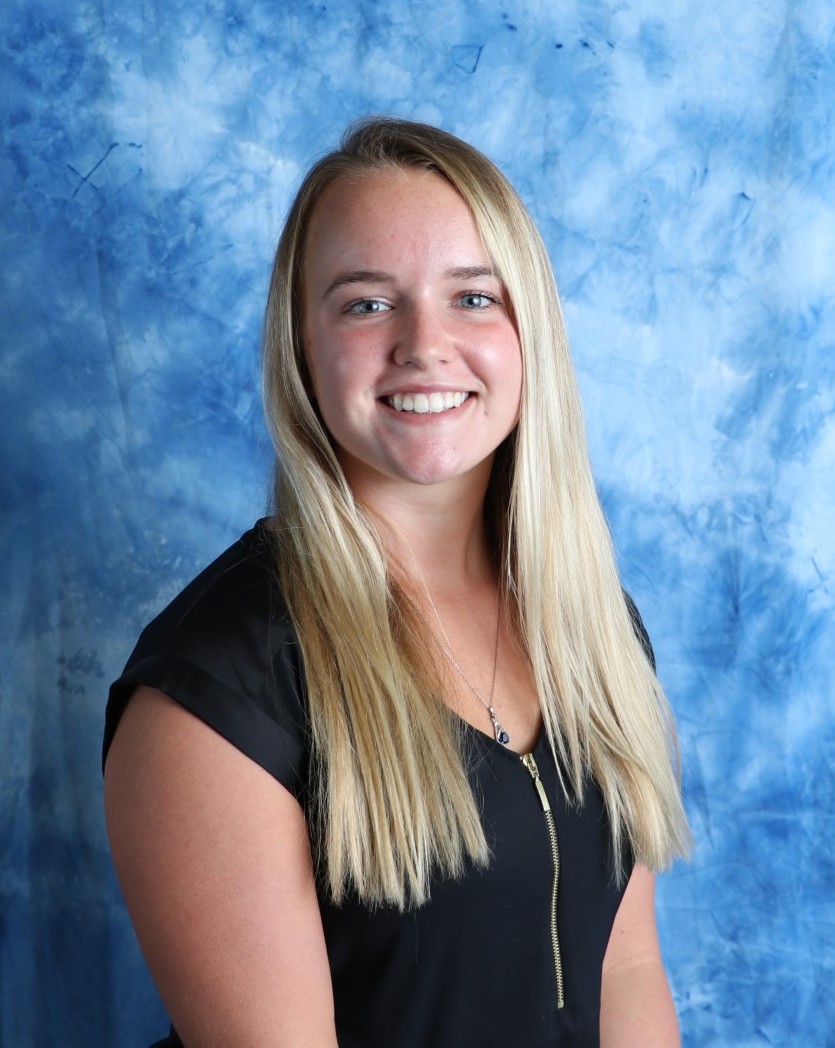
Laura Fischer (P2)
Project: “Open Data and Model Fitting with AMPL”
What appealed to you about the ATOM internship?
I applied to the ATOM internship because I wanted to gain a better understanding of machine learning and how it can be used to impact healthcare. I had learned a little bit about it in my Biotechnology class, but I thought the hands-on approach would help me get a deeper understanding. I thought this would be a cool way to improve my computer skills while experiencing a research-based, nontraditional career path for pharmacists. I also was interested in ATOM’s goal of speeding up the timeline of drug development, and I wanted to see how they used Machine Learning technology to work toward that goal.
Tell us about the experience.
My internship primarily consisted of writing and modifying Python code to work with public datasets and build machine learning models through the ATOM Modeling PipeLine (AMPL). I was working with four gene targets, training models to predict PIC50 values for them. The most accurate models can now be used to predict activities of new, unresearched compounds.
What fascinates you most about the relationship between pharmacy and machine learning?
I was really fascinated to see the actual impact that machine learning can have on pharmacy, and healthcare in general. I never thought I’d have a hands-on experience working directly with data science, so it was really cool to see how this makes an impact on the drug development process.
***
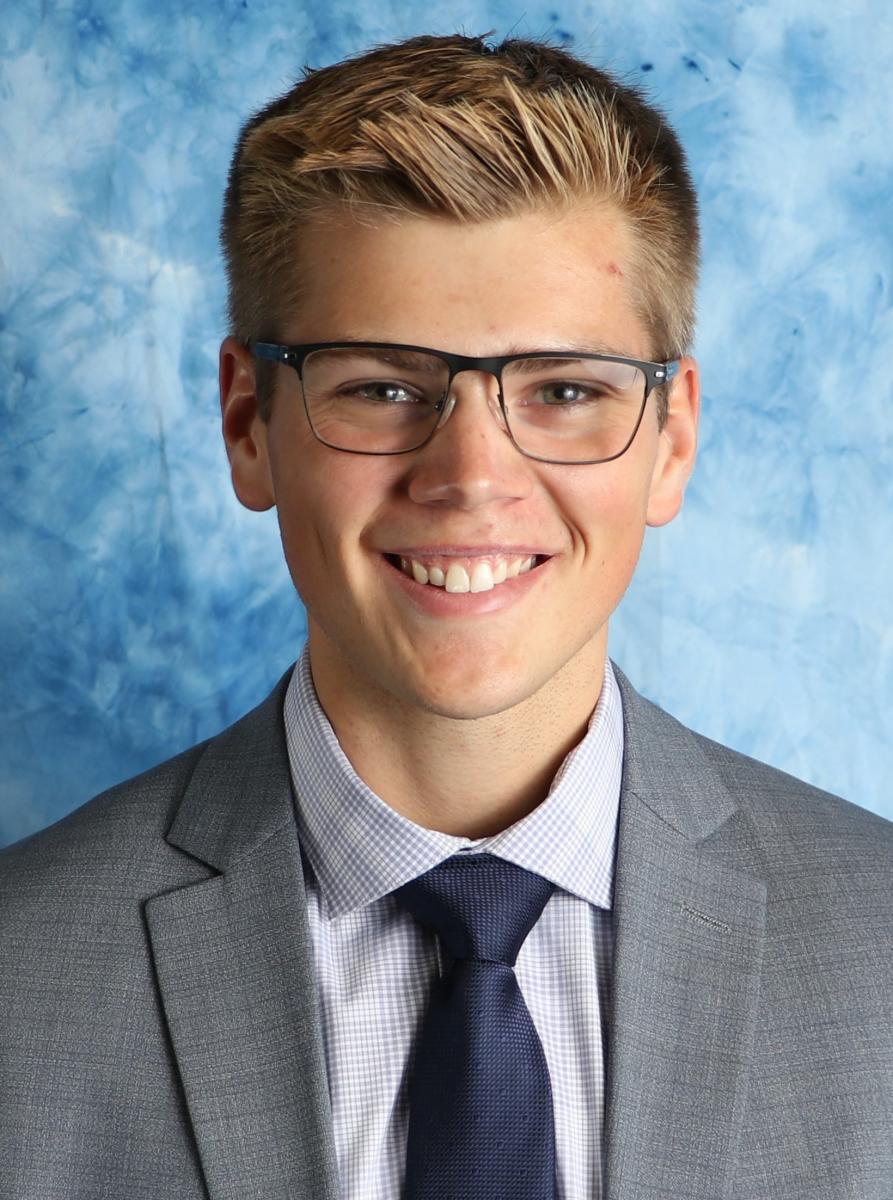
Logan Van Ravenswaay (P2)
Project: “Visualize Data: A Python Function to Generate Interactive Plots and Accelerate Exploratory Data Analysis”
Why did you choose to pursue pharmacy?
I always knew I wanted to pursue a career in healthcare, but I struggled with choosing a path. I loved my chemistry and biology courses in high school, so I thought pharmacy would be the perfect blend of the two.
Why did you decide to apply for the ATOM internship?
I applied for the ATOM internship because it would involve blending a computer science-based approach with drug discovery. I wanted to learn more about the drug discovery process and how we can improve it. However, both subjects were very much outside of my wheelhouse. I was excited by the challenge, as well as how I would be able to take what I learned with ATOM and use it to launch a potential career in drug discovery.
What fascinates you most about the relationship between pharmacy and data science?
I came into this internship with very little knowledge on computer science and how it might impact the future of drug discovery. However, I cannot be more excited about this relationship between machine learning and pharmacy. My time with ATOM has shown that data science is an integral piece of drug discovery. The sheer amount of potential therapeutic compounds far exceeds our ability to select drug candidates by hand. ATOM’s modeling tool and others like it can accelerate this discovery process, as well as be adapted to choose the best drug for a particular patient.
***
Connor Miller (P3)
Project: “Working with Open Data Sources: PK-DB, Lombardo Dataset, and AstraZeneca”
Why did you pursue pharmacy?
I enjoy the blending of math and science that can be found in pharmacy. Pharmacy also offers an opportunity to provide health services and benefit patients without being as “hands-on” as other providers, such as physicians or physician assistants. I find it amazing that drugs are just these small molecules that can have substantial and even life-saving effects on the body and its chemistry.
Tell us about your experience with the ATOM internship.
The overarching goal for my project was to help advance ATOM’s work with open source data, which can be more widely shared with the public compared to proprietary datasets. Typically, a larger dataset will result in machine learning models with better accuracy or more predictive power, so finding open source datasets is important in the effort to build these models.
What fascinates you most about the relationship between pharmacy and data science?
I think the relationship between pharmacy and data science will become increasingly important in the future, particularly in the area of drug development. Through machine learning models, companies in the pharmaceutical industry will be able to much more quickly identify compounds that may be effective at a certain target, or screen out compounds that are likely to have toxic effects. What excites me the most about this is that new treatments may be found and developed at a faster rate, thanks to these advances in data science and machine learning.
What have you learned from this experience?
Starting from knowing very little about coding or research, I have been able to learn a lot through this experience in terms of technical skills. I was also able to gain experience working with a virtual team. Despite the fact that we were all working from home, we were able to still have good communication. I am so glad that I was able to take part in this opportunity, and I found it to be an incredibly enriching experience in my pharmacy education.
Media Contact:
Katie Grieze
News Content Manager
kgrieze@butler.edu
260-307-3403